Most attempts to harness AI tools fail. Despite massive hype around the new systems entering the market, most deployments fail due to common pitfalls. Mikkel Rodam at babelforce addresses the 3 biggest traps and how to avoid them.
I’m a salesperson who makes their living selling AI. I’m invested in making AI look good. So you can trust me when I say that the failure rate for AI projects is enormous.
Only one-fifth of AI projects go live and fulfill their intended function.
That’s the bad news.
The good news is that the reasons they fail are clear – and you can easily avoid those failures yourself.
So I’m going to outline the 3 biggest points of failure for new AI projects. But if I was going to summarize this article, I would say:
- AI isn’t magic.
- It still takes work to get started.
- Sometimes humans are best.
Ok – time for the problems and solutions.
Problem #1 – When AI Isn’t Ready for the Frontlines
When you see funny AI stories in the press it’s almost always a Generative AI (GenAI) solution going off-script.
My favorite example at the moment is the Savey Meal Bot. The idea was simple: you tell the Meal Bot what ingredients you have, and it provides a recipe.
The problem? Users asked for recipes incorporating bleach, soap, medicines, and all kinds of dangerous items. The Meal Bot, of course, played along and advised users to consume poison.
That’s a standard “guardrails” problem; the Bot wasn’t told to avoid non-food items. The developers have fixed this now. Users can only choose ingredients from a pre-approved list.
However – there is nothing to stop users selecting “anchovies, blue cheese, custard, and papaya”. The Meal Bot will still suggest a recipe. Just not one you’d want to eat.
This shows us that, even with guardrails, there is plenty of scope for error.
Solution – Human in the Loop + Conversational AI
Many experts are hesitant about GenAI in frontline service. The chances of a ChatGPT-style tool getting “creative” are too high.
Conversational AI, meanwhile, is a far more predictable option. Unlike GenAI, which can create “new” content, Conversational AI is focused on comprehending queries and finding the right data from a set.
Conversational AI is like: a well-trained agent who knows your processes inside out.
GenAI is like: a creative but unpredictable agent who never did the training at all.
There are still exciting use cases for GenAI, but the business-ready ones are “Human-in-the-loop” i.e. supervised in some way. In particular, GenAI tools that support and inform agents are a major opportunity for call centres. According to IBM, 94% of surveyed businesses acknowledged enhanced agent productivity as a key benefit.
Meanwhile, 90% of consumers place more trust in businesses when its AI solutions are human-in-the-loop.
Problem #2 – When AI Is Siloed
One of the longest running problems in customer service is siloed systems. Close to 60% of contact centre leaders still say communication silos and system complexity hurt their CSat.
The question any team should ask when buying software is: are we buying another silo?
And yet AI systems are creating new silos daily.
The current AI hype means that a lot of businesses are buying solutions which are pretty much “plug and play”. You turn on the chatbot or the VoiceBot and it *seems* to start working right away.
You may even get a few helpful use cases without any serious integration. For example: if your store opening hours are available on your website, almost any AI tool will be able to help customers who ask the question “what are your opening hours?”
But that only helps you with customers who have generic questions about your business. What about customers whose questions are about their relationship with your business?
The most common and the most valuable queries a business gets are things like: “where is my order” / “I need help understanding my bill” / “I want to renew my contract”.
Solution – Integrate AIs as Deeply as Any Other Tool
The only way for any AI tool, however brilliant, to help with those queries is to be integrated via APIs with systems like your Helpdesk, CRM, and other key systems.
This is a fundamental part of the equation which is very often overlooked. The point is this: however impressive an AI tool is, it only knows what somebody tells it. For ChatGPT that means what it can find on the internet. For a Conversational AI system in your customer service, that means what it can find in your Systems of Record.
Depth of integration is directly proportional to achievable value.
Problem #3 – When AI Blocks Access to Humans
Here’s where I might lose you… but it is a problem if your AI and automation projects block access to human agents.
I know – reducing human labor is the whole point, right?
But this is something you need to be careful with. You have customers who will never use automated services. You have customers who are happy to use automation, some of the time. You also have a lot of customers whose query means automation isn’t the appropriate strategy.
Solution – Scrap the “All or Nothing” Approach to AI!
Take the case of major German energy company I work with. They’ve put a lot of time and effort into their Voicebot for a saving of half a million Euros annually.
But what’s really interesting is where those savings come from.
- 5% of volume – Fully automated interactions. Simple or low-priority conversations which the Voicebot can solve easily.
- 20% of volume – Partially automated interactions. Often one simple query, solved in automation, followed by a warm handover to an agent for a more complex query.
- 55% of volume – Light-touch automation. Cases like IDing the customer and gathering caller intent.
In total, 80% of the business’s interactions involve their Voicebot. It’s a huge reduction in cost and handling time. But only 5% of customers actually avoid human agents.
For other clients the number is higher (or lower) depending on factors like their common query types and average deal value. But in general, 5% is pretty representative.
So when I hear claims about huge call containment numbers with AI, I get suspicious. It is rarely feasible to fully automate such a wide array of queries but, more importantly, it is rarely desirable to do that.
And if there is one especially big way to fail with AI it is: setting a goal you don’t really want to achieve!
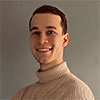
Mikkel Rodam
Mikkel Rodam is an Enterprise Account Executive with babelforce. He will be talking more about common AI pitfalls on September 19 in Stockholm, alongside speakers from Zendesk and Happirel.
You can book your place for this free event here
For more information about babelforce - visit the babelforce Website
Call Centre Helper is not responsible for the content of these guest blog posts. The opinions expressed in this article are those of the author, and do not necessarily reflect those of Call Centre Helper.
Author: babelforce
Reviewed by: Rachael Trickey
Published On: 10th Sep 2024 - Last modified: 22nd Oct 2024
Read more about - Guest Blogs, babelforce, Mikkel Rodam