Kenneth Brisco of NICE introduces us to churn analysis to predict and prevent customer attrition.
Customer churn can be extremely taxing on any organization. The toll it takes is not limited solely to lost revenue.
Statistics show that, on average, 65% of an organization’s business comes from existing customers, and acquiring new ones is 15 times more expensive than retaining existing customers.
So the full cost of customer churn consists of lost revenue and the marketing costs involved with replacing churned customers with new ones.
The trouble is, retaining customers has historically been a lagging business indicator: it’s only after organizations have lost customers that they would assess what went wrong.
But with increasing access to data, the opportunity exists for organizations to leverage predictive churn analysis capabilities to identify at-risk customers and proactively prevent them from leaving.
The ability to predict that a particular customer is at a high risk of churning – while there is still time to proactively address the situation – represents a great opportunity to increase customer loyalty and avoid loss of revenue.
How Customer Churn Analysis Works
Customer churn analysis can be accomplished by fitting statistical models to historical data and trying to find a pattern in customers that may result in churn.
Forward-thinking organizations are leveraging artificial intelligence (AI) and machine learning to forecast future trends and behaviors and identify previously hidden indicators that help to predict churn.
There are four major steps in the process:
- Data gathering and preparation
- Building the predictive model
- Testing and validation of the model on real customers
- Continually updating the model as the process repeats itself and the churn rate reduces
While simple in theory, the realities involved with achieving this proactive retention goal are extremely challenging.
Using Customer Journey Analytics to Predict Churn
Churn prediction modeling techniques attempt to understand the precise customer behaviors and attributes which signal the risk and timing of customer churn.
Customer journey analytics meets that criteria by combining data across multiple touchpoints to understand how customers navigate through brand interactions over time.
This allows organizations to analyze customer journeys and identify behavior that implicitly indicates that a customer is happy or dissatisfied with the service they just received.
The importance of using journey analytics is that it evaluates both past and present journeys to predict the likelihood of churn. For example, suppose that a customer contacts a call centre to cancel their subscription.
The reason why the customer churned may not be found at the touchpoint in which the churn occurred. It might be hidden in journeys from their previous interaction history.
With full visibility into the entire journey, we find that the customer received an unusually high phone bill and initially contacted a call centre to adjust the payment.
Although it is a common journey, in some cases it might trigger a complaint or even churn.
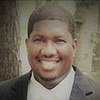
Kenneth Briscoe
Within the current state of customer service, it is no longer enough for organizations to only react to churn. Prediction and prevention of customer churn requires a holistic approach to advanced analytics that provides further understanding into the customer journey.
Organizations that effectively utilize customer journey analytics to reduce churn will not only improve the customer experience but also differentiate their brand from competitors.
This blog post has been re-published by kind permission of NiCE – View the Original Article
For more information about NiCE - visit the NiCE Website
Call Centre Helper is not responsible for the content of these guest blog posts. The opinions expressed in this article are those of the author, and do not necessarily reflect those of Call Centre Helper.
Author: NiCE
Published On: 25th Sep 2019
Read more about - Guest Blogs, NiCE