Fabrice Martin of Clarabridge shares nine ways of analysing customer data to improve the overall customer experience.
Customer data comes from all directions, from many sources, and in dozens of formats.
Omni-source data collection can aggregate it and customer analytics identifies trends that illuminate the overall customer experience.
But to understand what’s really happening with customers, it’s crucial to dig deep into the data.
Here are nine examples of analysis techniques to find essential insights.
1. Detect New Themes Using Machine Learning
One of the benefits of analysing unstructured text is the chance to discover completely new or surprising topics in the feedback.
Machine learning techniques can identify these new patterns automatically, shining a spotlight onto new areas of interest as they occur in real time.
Social media data is a great example because themes of feedback occur constantly.
For example, a car manufacturer sees new complaints about bumpers—which were fine at the factory. They trace them back to how bumpers are stacked for shipping and remedy the situation quickly.
2. Monitor Trends and Spikes in Themes
It’s important to view overall volumes of feedback, but also to see how they shift over time.
Trending data allows analysts to see movement in theme volumes by days, weeks, years, or even in a small 24-hour period.
Spikes in data also provide an opportunity to quickly react to customer trends or monitor ongoing issues to gauge their importance.
If a campaign is launched that prompts negative reactions and these outweigh the positives, it can be stopped quickly.
3. Find the Root Cause
It can be difficult to find the root cause of a spike or trend when customer volume, sentiment, or satisfaction scores start moving.
This difficulty is multiplied as more data sources are thrown into the mix, and when several layers of structured attributes may be contributing to the change.
Using statistical analysis techniques, multiple attributes, themes, and natural language understanding (NLU) attributes (for example topics, sentiment, emotions and effort) can be analysed at once.
Then the significance of these factors can be measured, unlocking the most important issues.
This type of analysis could show, for example, that the root cause of low sentiment for a car dealership is that its new, purpose-built servicing centre has no wi-fi for while-you-wait-customers and no public transport links nearby so it can be accessed easily for car pick-up.
4. Segment to Identify Key Differences
Segmenting data makes it easy to find drivers of customer satisfaction among different groups.
Consider: what themes do high-level revenue customers like more compared to lower-revenue customers? And which of these themes do high-value customers mention most often?
Analysing different segments of data using the same models enables points of differentiation to be located.
5. Use KPI Metrics in Conjunction With Unstructured Themes
Use of KPI metrics such as review rating, Customer Satisfaction scores (CSat), or Net Promoter Score (NPS) is common.
However, additional insights can be found by mapping these metrics against unstructured feedback to reveal the ‘why’ that is driving those scores.
A leading managed cloud provider, for example, runs two survey questions, one NPS and one open-ended on which they run theme detection. This allows the company to determine the key drivers of the NPS score, in other words the ‘why?’, far more effectively than if they had asked fifteen questions.
As a general principle, just remember that shorter surveys will invariably elicit higher response rates.
6. Apply Sentiment Analysis to Find Areas of Customer Unhappiness
Sentiment analysis is an incredibly useful tool in analysing all sources of unstructured data. It examines data for positive or negative context, providing an additional dimension of analysis beyond volume or KPI metrics.
Sentiment analysis also illustrates how different themes affect customer experience, either positively or negatively.
Additionally, sentiment can act as a singular metric of performance across all sets of data.
7. Pair Sentiment Analysis With CSat Metrics
Looking at KPI metrics in conjunction with themes shows the high- and low-performing touchpoints along the customer journey; however, it does not always show how to improve those metrics.
It might be that long call waiting times produce low sentiment—but they are also associated with average CSat scores. This indicates that although people are unhappy with call waiting, it doesn’t significantly impact their overall customer experience.
Equally, low sentiment regarding website navigation is also associated with low CSat, so improving the website is likely to generate improvements to the overall CSat score.
8. Create Predictive Models
Predictive modelling techniques can help guide business decisions and predict improvements in KPI metrics or in monetary metrics like revenue.
When done correctly and when using a combination of structured and unstructured data inputs, predictive analysis can be a confidence booster for customer experience investments.
A global drinks manufacturer, for example, could track social mentions of different sweeteners to predict reactions to new products and understand brand impact.
9. Combine All Techniques to Create a Data-Driven Culture
Data analysis can be incredibly powerful, but its value will only be fully realized if action is taken on the insights that are uncovered.
Dashboards, alerts, and case management functionality can be used to push data and insights through the organization.
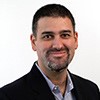
Fabrice Martin
These tools allow information to be delivered to the right person at the right time to take action, opening communication across departments and at all levels of leadership.
The volume of customer feedback will never slow down—organizations must learn to absorb, react, and learn from data faster and more efficiently than ever.
Thanks to Fabrice Martin, the Chief Product Officer at Clarabridge, for sharing this article with us.
Author: Robyn Coppell
Published On: 22nd Aug 2019 - Last modified: 27th Aug 2020
Read more about - Customer Service Strategy, Clarabridge